Why Manual Effort Still Dominates the Data Science Lifecycle—and How to Change It
Recent Posts
Despite the wealth of tools available today, much of the data science process is still bogged down by manual labor. According to our survey with Techstrong Research and Databricks, key tasks like data access, feature engineering, and model deployment still require significant hands-on effort. This isn’t just slowing things down—it’s holding back the potential for rapid AI-driven innovation. Let’s explore why this happens and how automation can flip the script.
Where Are Manual Processes Slowing Us Down?
The data science lifecycle is complex, and many stages still rely on manual work. Our survey highlighted the biggest pain points:
- Data Access and Management: Finding and accessing the right data is a major roadblock—23% of respondents said it’s their biggest challenge. With scattered, siloed data sources, data scientists spend far too much time hunting down and wrangling data instead of analyzing it.
- Understanding Data Semantics: Another 20% of respondents said their top headache is understanding data from different sources. The variation in meanings, formats, and quality means data scientists are stuck manually cleaning and aligning data to make it usable.
- Feature Engineering: Arguably the heart of the AI/ML lifecycle, creating effective features is an iterative, resource-heavy process. It requires both domain expertise and data science skills—and it’s one of the most time-consuming, manual tasks in the cycle.
- Deployment and Maintenance: Even when the model is ready, getting it into production isn’t always easy. Manual testing, configuration, and ongoing maintenance slow everything down, preventing businesses from getting the most out of their models quickly.
The Hidden Costs of Manual Work
These manual processes are more than just an inconvenience—they’re costly. Here’s how:
- Lengthy Development Cycles: Manual tasks add months to development. In fact, 35% of professionals surveyed said their model development cycles last over six months—far too slow to compete effectively in today’s fast-moving markets.
- High Skill Requirements: Because manual work demands specialized knowledge, scalability is limited, and teams become overly reliant on niche expertise.
- Operational Bottlenecks: Lack of automation means bottlenecks in updating and retraining models, which can stifle innovation and efficiency.
The Move to Automation
The path forward is clear: to unlock predictive AI’s true potential, businesses need to automate. Here’s how to get started:
- Adopt End-to-End Predictive AI Platforms: Automating repetitive tasks like data preparation and feature engineering can dramatically speed up development. Platforms like FeatureByte help automate these stages, so data scientists can focus on building smarter models, not on tedious tasks.
- Use a Data-First Approach: Automation tools that quickly identify and classify data remove the need for manual data prep. With a data-first platform, teams can spend less time searching for the right data and more time putting it to work.
- Leverage Automated Feature Engineering: Automating feature generation reduces reliance on domain experts and fast-tracks the modeling process. It not only boosts productivity but also enables non-data scientists to contribute to the process.
The manual grind in AI/ML development is a massive bottleneck. By embracing automation across the data science lifecycle, businesses can slash timelines, break down bottlenecks, and focus on delivering faster, real-time value. The less time spent on manual intervention, the more predictive AI can become the engine for agile, data-driven decision-making.
Ready to streamline your data science process? Download our full report with Techstrong Research and Databricks to learn more.
Explore more posts
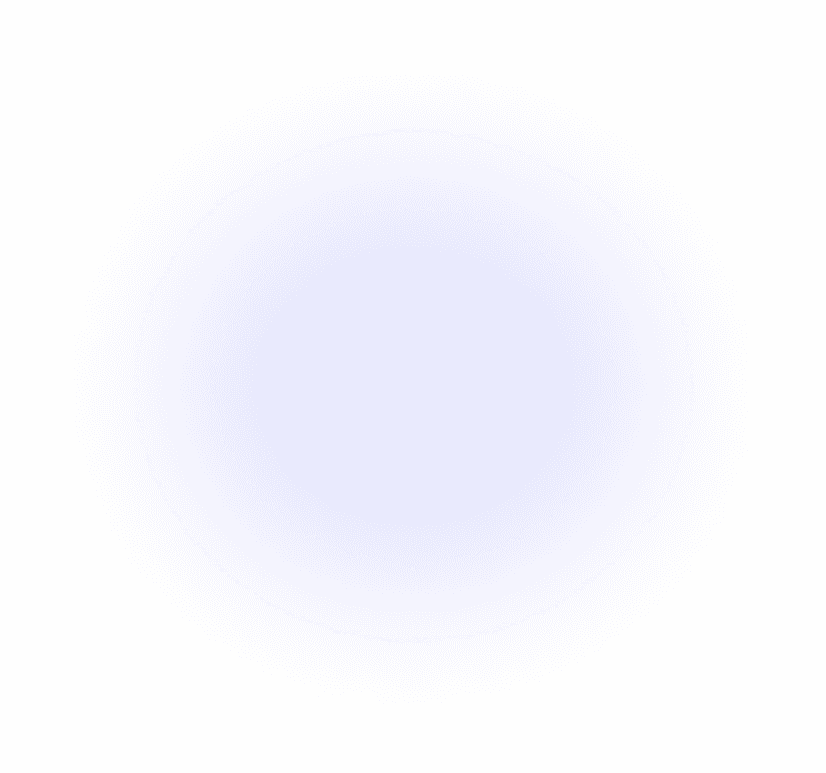