Why Speed Matters: The Impact of Long AI/ML Development Timelines
Recent Posts
The value of AI and machine learning in business decisions and operations cannot be overstated. A whole generation of companies’ business models are based on an AI/ML foundation. But there’s one major roadblock stopping many companies from reaping the benefits: time. According to our recent survey report with Techstrong Research and Databricks, the lengthy process of developing and deploying AI/ML models is a serious obstacle to achieving business impact.
The Problem: Long Development Timelines
One of the biggest challenges in AI/ML projects is how long it takes to go from idea to implementation. Our survey revealed:
- 35% of respondents said their AI/ML projects take over six months to reach production.
- Another 19% reported timelines between four to six months.
These long cycles are more than just a headache—they’re a missed opportunity. When AI models take months to build, companies lose the chance to act on critical market shifts, streamline operations, or make smarter decisions in real time.
The Cost of Delays
The consequences of these delays are clear. According to the survey:
- 28% reported significant losses in business opportunities.
- 33% of respondents said delayed AI/ML development moderately impacts their business.
Predictive AI/ML is meant to boost decision-making and outcomes. But when models take over half a year to deploy, the insights are often stale by the time they’re ready. In a fast-evolving market, speed is everything.
The Solution: Shorter Development Cycles
To tap into the true power of AI/ML, businesses need to reduce the time from problem to deployment. Here’s how:
- Automated Data Prep & Feature Engineering: Manual data preparation and feature engineering eat up a lot of time. Automating these steps lets data scientists spend more time on experimentation and innovation.
- End-to-End Integration: Instead of juggling disconnected tools, companies should use platforms that streamline the entire AI/ML lifecycle, reducing handoffs and bottlenecks.
- Better Collaboration: It’s not just about the tools—people matter way more. Aligning data scientists, engineers, and domain experts can drastically cut down on delays.
The Power of Automation
The biggest roadblock in AI/ML is the time it takes to go from concept to deployment. To turn AI into a strategic advantage, companies need to embrace automation and integration, speeding up the process so they can capitalize on opportunities while they’re still hot.
Want to learn more? Download our full report with Techstrong Research and Databricks to see how automation can accelerate your AI/ML journey.
Explore more posts
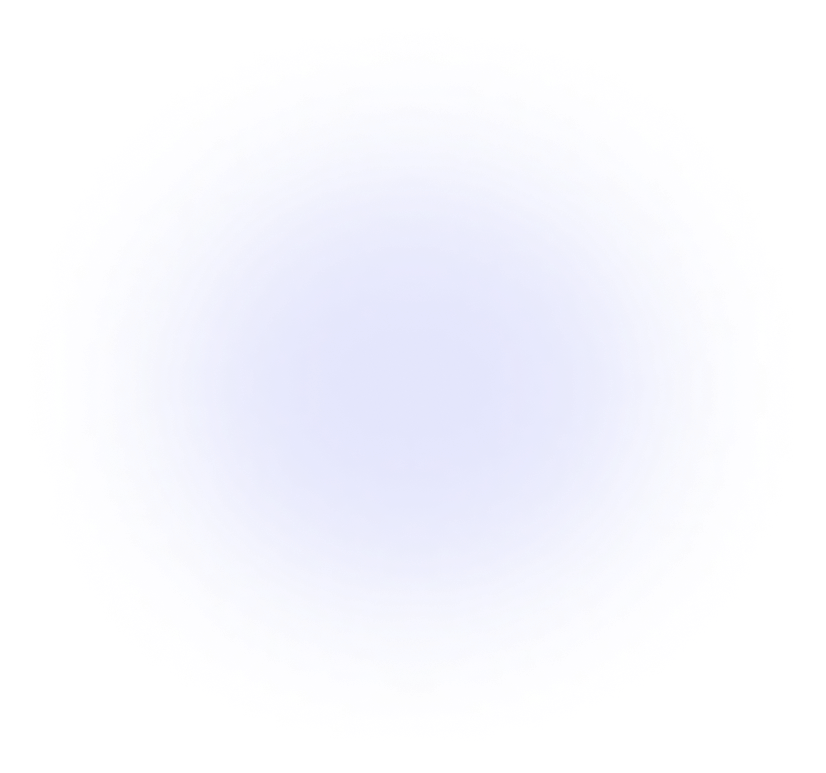